Education
Discriminant Analysis – Things You Must Know About It
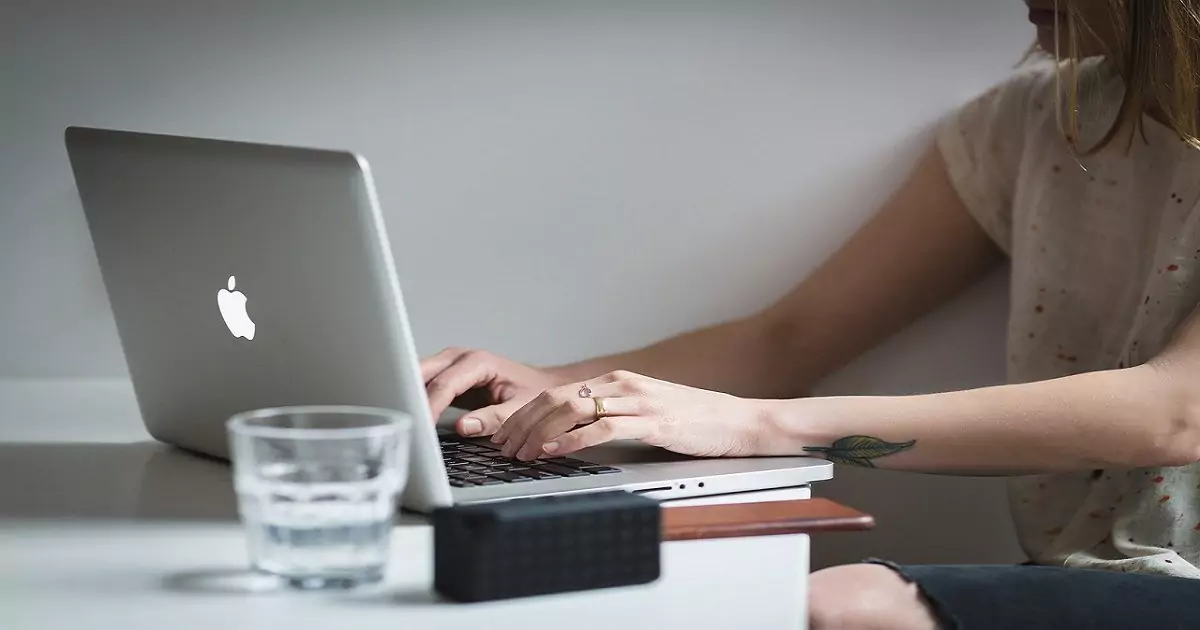
Knowing things essential to conduct a discriminant analysis is important, especially if a researcher gets stuck in classifying large data sets into different manageable groups. Thus, this article aims to explain discriminant analysis by describing its importance, types and 6-step procedure.
Introduction:
Discriminant analysis is a statistical method mostly use in market research which aims to classify observations into different categories or groups. Stated in another way, discriminant analysis is important as it helps researchers in assigning objects to a group among a number of known groups. As a matter of course, it is simply a statistical technique that classifies observations into groups based on scores of one or more quantitative as well as predictable variables. For instance, if a doctor wants to classify a patient into low or high-risk groups for stroke, then the best tool that will be extremely useful for the purpose is discriminant analysis. This analysis will help the doctor classify patients into a low and high-risk group based on personal attributes, such as body weight, cholesterol, lifestyle behaviour, and patient family history.
Importance Of Discriminant Analysis:
There is a long list of benefits of using the discriminant analysis process. Firstly, it helps in determining predictor variables that can easily be related to dependent variables. Secondly, it is also very important to predict certain facts about the dependent variables. Thirdly, market researchers use this analysis method in the perpetual mapping creation. Fourthly, it involves the use of a perceived distancing technique that differentiates this method from other classical statistical analysis methods.
Apart from all previously mentioned benefits, another important quality of discriminant analysis is that it can also be used in combination with other statistical analysis methods, such as cluster analysis. In a nutshell, discriminant statistical analysis is important if the purpose of research is to determine the effect of the predictor variables on dependent variables, predict facts about the dependent variables, classify subjects into different groups based on personal properties, take into account the perceived distancing technique, and create perpetual maps for marketing research.
Types Of Discriminant Analysis:
Before deciding whether this analysis will be useful for a research proposal or whether other options need to be considered, it is important to know about different types of discriminate statistical analysis. Basically, this statistical method can be divided into four major types:
Linear Discriminate Statistical Analysis:
The linear discriminate statistical analysis is the simplest form of analysis. Its sole purpose is to reduce the number of available features before starting classification. It is mainly used in machine learning and market research, where the linear combination for specifications is differentiated based on two or more objects and events. Furthermore, the linear analysis can be performed with and without a stepwise selection of variables.
Multiple Discriminate Statistical Analysis:
This type of analysis is slightly difficult to perform as compared to linear and canonical analysis methods. This is because it aims to find discriminant functions that can minimise differences within groups and maximise differences between different groups with the use of canonical functions. In simple terms, the multiple discriminate statistical analysis is helpful in compressing the multi-variate signal, so that a low dimensional signal or category can be produced.
Quadratic Discriminate Statistical Analysis:
Unlike other types of analysis, this analysis classifies observation in the form of groups that has the least squared distance. Furthermore, in this method, researchers are not allowed to reduce the squared distance into linear functions.
Canonical Discriminate Statistical Analysis:
The Canonical discriminate statistical analysis or CDA is a multi-variate technique important to determine relationships between a set of independent variables and categorical variables. It includes canonical correlation and Principle Component Analysis in order to perform the dimension reduction.
Discriminant Analysis Is A 6-Step Procedure:
Following the brief description of steps vital to performing discriminant analysis for research:
Select A Research Problem:
In many cases, a research problem is a statement, a question, or a claim to be verified by choosing an extensive research process. However, for a research problem to be analysed by discriminant analysis, it must contain more than one variable that needs to be classified for extracting inferences. This step also includes the selection of objectives, evaluation of group differences on a multi-variate profile, categorisation of factors into different groups, and finding dimensions of discrimination within and between different groups.
Select Research Design Issues:
Issues in designing a research may include the identification of variables whose correlation or interconnectedness needs to be calculated via the research process. Thus, the selection of independent variables, calculation of sample size, creation of analysis, and holdout samples are the major tasks necessary to perform at step number 2 of this analysis process.
Review Assumptions:
Reviewing assumptions and setting research as per its requirement is the fourth step in discriminant statistical analysis. Some of these assumptions include linearity in relationships among variables, normality of independent variables, selection of equal dispersion matrices, and lack of multi-collinearity. Once the researcher has confirmed that the independent, as well as dependent variables, fulfil all these assumptions, then the process of discriminant analysis can be performed.
Estimate Discriminant Functions:
It is the stage of finding discriminant functions and their significance. Furthermore, the determination of optimal cutting scores, criteria for accessing hit ratio, and calculation of statistical significance of predictive accuracy can further help in estimating discriminant functions and their significance.
Interpret Discriminant Functions:
Interpretation of discriminant functions refers to knowing what discriminant functions tell about the relationship and classification of variables into different groups.
Validate Discriminant Results:
Last but not least is the step to validate all findings of the discriminant analysis process. Unlike other types of analysis processes, techniques important to validate results of the discriminant analysis include ‘split-sample or cross validation’ and ‘profiling group difference’.
Concluding Remarks:
In a nutshell, discriminant analysis is a type of statistical analysis important to classify different objects into different groups based on their personal properties. This type of analysis can be applied to any type of research project, including marketing, medical, engineering, public relationships, mathematics, and many more. Its types include linear discriminate statistical analysis, multiple discriminate statistical analysis, canonical discriminate statistical analysis, and quadratic discriminate statistical analysis. In the end, by following the above mentioned six simple steps, discriminate analysis can easily be applied to any research project. However, if you are facing any issues in conducting this analysis, you must seek help from Dissertation Writers UK.
Read more about – How To Improve Your Writing Skills
Education
The Ultimate Guide to Choosing Between Rich Text vs Plain Text
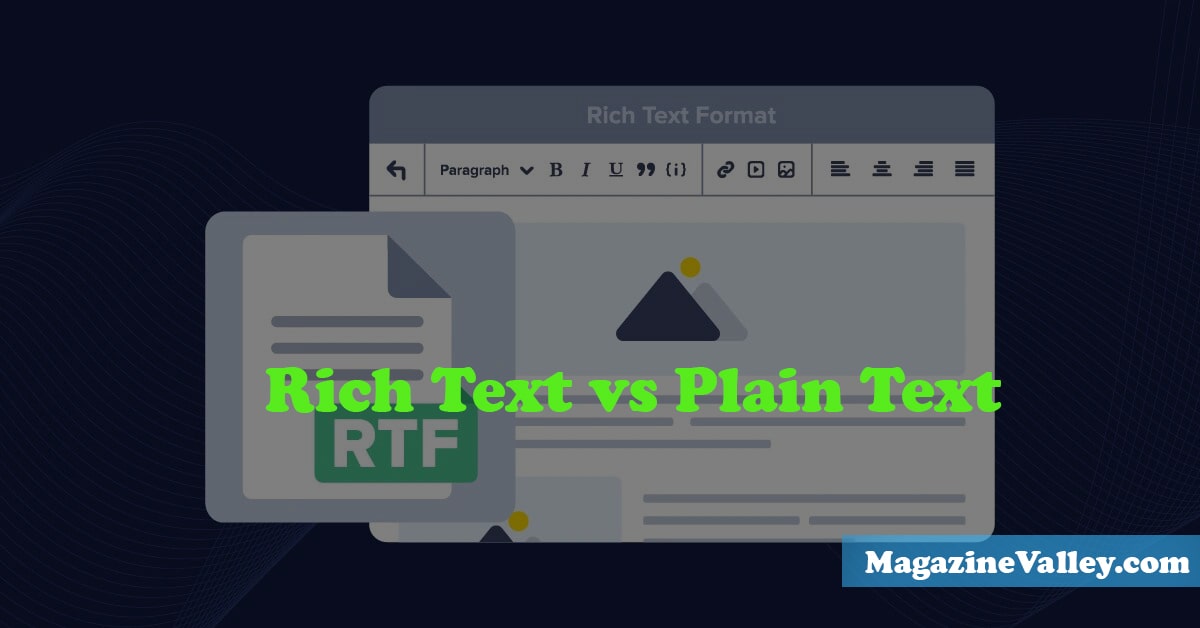
Are you a text aficionado looking to level up your writing game? Are you torn between the simplicity of plain text and the allure of rich text formatting options? Well, fret not! In this ultimate guide, we will delve into the world of Rich Text vs Plain Text, exploring their differences, advantages, best use cases, and common pitfalls to help you make an informed choice. So buckle up as we embark on this exciting journey through the realm of text formats.
Understanding the Difference between Rich Text and Plain Text
Rich Text and Plain Text may seem like two sides of the same coin, but they cater to different needs in digital communication. Rich Text offers many formatting options, allowing users to add colors, fonts, styles, and multimedia elements like images or hyperlinks to enhance their content. On the other hand, Plain Text strips away all fancy embellishments, presenting information in a simple and straightforward manner without any additional formatting.
While rich text is visually appealing and can help highlight key points or create eye-catching designs, Plain Text shines in its simplicity and compatibility across various platforms. Understanding when to use each format can significantly affect how your message resonates with your audience. So, whether you opt for the elegance of rich text or the no-nonsense approach of Plain Text ultimately depends on your specific communication goals.
Advantages of Using Rich Text
Rich text offers a wide range of advantages that can enhance your documents’ overall appearance and functionality. One major advantage is the ability to format text in various styles such as bold, italic, underline, and different font sizes. This lets you emphasize important information and make your content more visually appealing.
Additionally, rich text editors often include features like bullet points, numbered lists, and paragraph alignment options. These tools help organize your content in a structured manner, making it easier for readers to follow along.
Another benefit of using rich text is the integration of multimedia elements such as images and hyperlinks. By incorporating visuals and interactive links within your text, you can create a more engaging experience for your audience.
Furthermore, rich text formats are compatible with most word processing software and online platforms, ensuring seamless document sharing and collaboration across multiple devices. Rich text’s flexibility and versatility make it a valuable tool for creating dynamic and professional-looking content.
Benefits of Using Plain Text
Simplicity reigns supreme when it comes to the benefits of using plain text. Plain text is clean and straightforward, making it easy to read and understand without any distractions or unnecessary formatting. This stripped-down approach can be especially useful when you want your content to be easily accessible across various platforms and devices.
Another advantage of plain text is its compatibility with different software applications. Whether sharing a document with colleagues or collaborating on a project, plain text ensures that your content will display consistently regardless of the program used. This universal readability can save time and prevent frustrating formatting issues.
Moreover, plain text files are typically smaller than rich text formats, making them quicker to load and easier to share. This efficiency can be particularly beneficial when working with large amounts of data or emailing documents.
The benefits of using plain text lie in its simplicity, compatibility, and efficiency – qualities that make it a practical choice for many writing tasks.
When to Use Rich Text vs Plain Text
When deciding between rich or plain text, it’s important to consider the purpose of your content. Rich text allows various formatting options, such as bolding, italicizing, and adding colors to enhance visual appeal. It is ideal for creating visually engaging documents like newsletters, brochures, or presentations.
On the other hand, plain text is best suited for simple and straightforward communication where formatting isn’t a priority. When sending emails or drafting legal documents that require clarity above all else, plain text can ensure that your message is delivered accurately without any distractions.
Consider your target audience when choosing between rich text and plain text. Rich text may be more suitable if you’re communicating with tech-savvy individuals who appreciate visual aesthetics. However, plain text might be the better choice if your audience values simplicity and efficiency in their reading experience.
Tips for Formatting and Editing Rich and Plain Text
When formatting and editing rich text or plain text, there are a few key tips to keep in mind. First, make sure to use headings and subheadings to organize your content effectively. This helps improve readability and makes it easier for readers to navigate through the text.
Additionally, pay attention to font styles and sizes when formatting rich text. Consistency is key here – stick with one or two fonts throughout the document for a clean and professional look. When editing plain text, focus on keeping sentences concise and clear. Avoid unnecessary jargon or overly complex language that could confuse readers.
Another important tip is to proofread your text carefully before publishing. Look for spelling errors, grammar mistakes, and formatting inconsistencies. Taking the time to edit thoroughly can greatly enhance the quality of your written content.
Consider using bullet points or numbered lists when presenting rich and plain text information. This can help break up long paragraphs into digestible chunks for readers who prefer skimming through content quickly.
Common Mistakes to Avoid in Using Rich and Plain Text
One common mistake to avoid when using rich text is going overboard with formatting. While it can be tempting to add multiple fonts, colors, and styles, it can make the text harder to read and distract from the content.
Another mistake in rich text is not checking for compatibility across different platforms. What looks great on one device may appear completely different on another, leading to a disjointed user experience.
A common error in plain text is neglecting proper spacing and line breaks. Walls of unbroken text can overwhelm readers and make important information hard to find.
Additionally, forgetting to proofread plain text carefully can result in typos or grammar mistakes that diminish the overall professionalism of your message. Always take the time to review before publishing.
Conclusion: Which One is Right for You?
Consider your specific needs and preferences when weighing the benefits and drawbacks of using rich text versus plain text. The rich text offers more formatting options and visual appeal, making it ideal for documents that require a polished presentation. On the other hand, plain text is straightforward and universally compatible, making it suitable for simple communication or coding purposes.
The choice between rich and plain text depends on your goals and requirements. Experiment with both formats to determine which suits your writing style and objectives best. Whether you opt for the versatility of rich text or the simplicity of plain text, mastering both formats will empower you to convey your message in any context effectively.
Education
Manguonmienphi Overview 2024
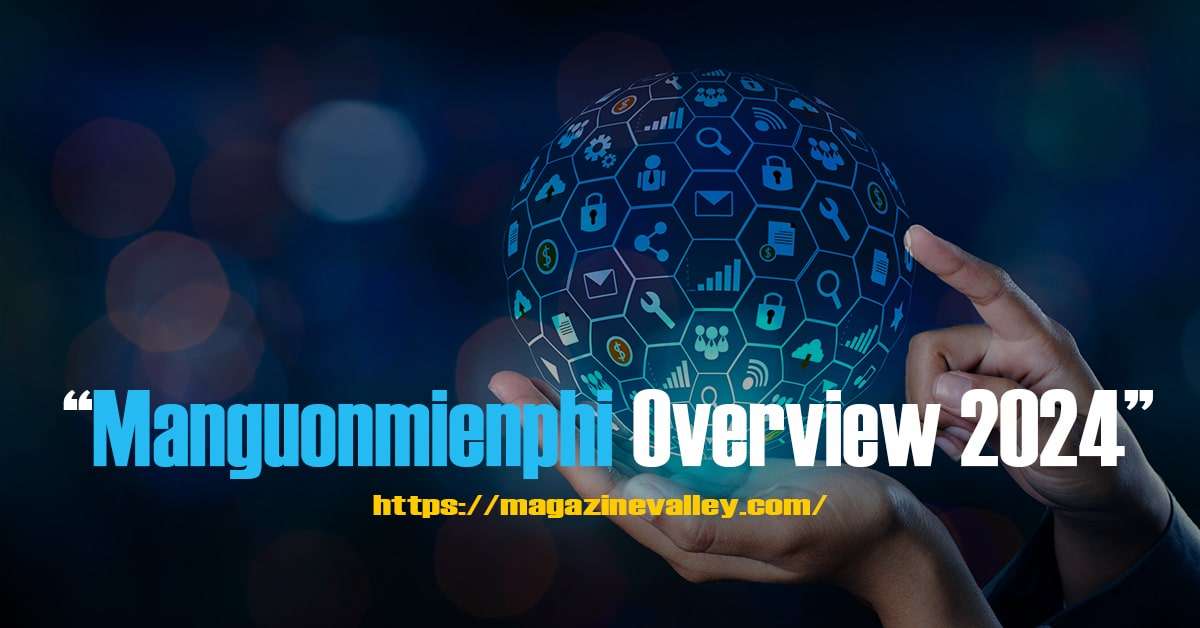
In the digital age, access to quality educational resources is paramount for learners and educators alike. Enter Manguonmienphi, a Vietnamese platform revolutionizing the way students, educators, and professionals access free, high-quality materials. From textbooks and articles to videos and more, Manguonmienphi is a treasure trove of knowledge designed to empower its users.
What is Manguonmienphi?
Manguonmienphi is an innovative platform dedicated to providing free educational resources. It caters to a wide audience, including students, educators, and professionals, offering a diverse range of materials that cater to different learning needs and preferences.
Comprehensive Resource Library
Whether you’re a student looking for textbooks or an educator seeking insightful articles, Manguonmienphi has you covered. The platform boasts an extensive library that includes:
- Textbooks: Covering various subjects and educational levels.
- Articles: Providing in-depth knowledge and research on a multitude of topics.
- Videos: Enhancing learning through visual and auditory means.
- More: Including guides, tutorials, and other valuable resources.
Features of Manguonmienphi
What sets Manguonmienphi apart from other educational platforms is its user-centric design and robust features that make learning accessible and enjoyable.
User-Friendly Interface
Navigating through Manguonmienphi is a breeze, thanks to its intuitive and user-friendly interface. Whether you’re tech-savvy or not, finding the resources you need is straightforward and hassle-free.
Powerful Search Engine
Manguonmienphi’s comprehensive search engine allows users to quickly locate specific resources. Simply enter a keyword, and the platform’s advanced algorithms will display the most relevant materials.
Personalized Recommendations
To enhance the user experience, Manguonmienphi offers personalized recommendations based on your browsing history and preferences. This feature ensures you always have access to the most relevant and useful resources.
Community Forum
Learning is more enriching when shared. Manguonmienphi’s community forum provides a space for users to connect, share ideas, and discuss various topics. It’s a vibrant community where knowledge is exchanged and collaboration is encouraged.
The Impact of Manguonmienphi
Manguonmienphi’s commitment to providing free and accessible education has garnered widespread praise. Its impact is particularly significant in Vietnam, where educational resources can sometimes be scarce.
Empowering Students
For students, Manguonmienphi is a game-changer. It offers an invaluable repository of resources that support their studies and foster a deeper understanding of various subjects. The availability of free textbooks, articles, and videos ensures that students have the tools they need to succeed academically.
Supporting Educators
Educators also benefit greatly from Manguonmienphi. The platform provides a plethora of teaching materials that can enhance lesson plans and offer new insights. Educators can also engage with the community forum to share best practices and collaborate with peers.
Bridging Educational Gaps
By providing free access to high-quality resources, Manguonmienphi helps bridge the educational gap, ensuring that everyone, regardless of their financial situation, has the opportunity to learn and grow.
Join the Manguonmienphi Community
Manguonmienphi is more than just a platform; it’s a community of learners and educators committed to the pursuit of knowledge. Whether you’re a student looking to excel in your studies or an educator seeking to inspire your students, Manguonmienphi offers the resources and support you need.
Education
Used as Campaign Talking Point Crossword Puzzle
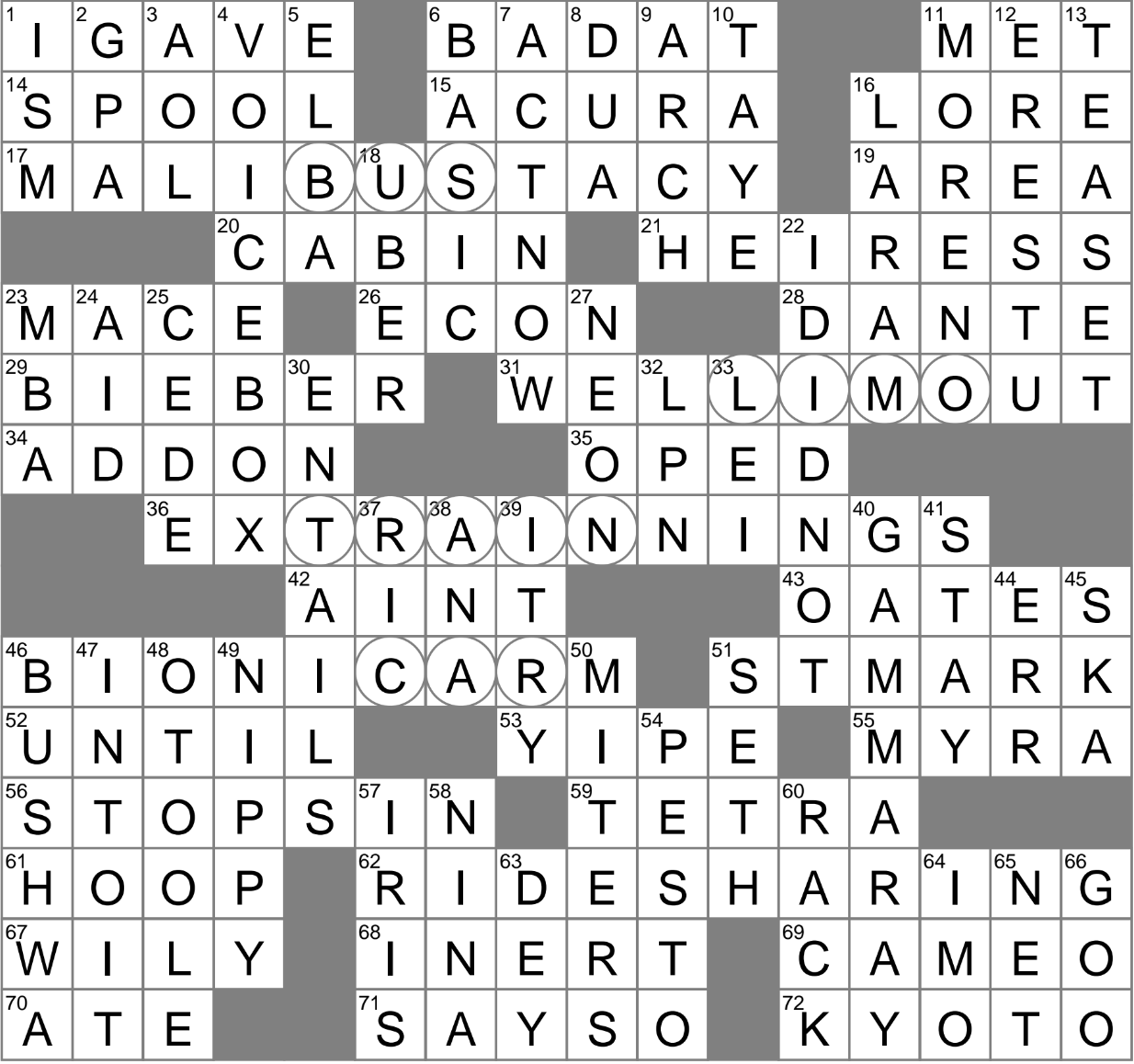
Used as Campaign Talking Point Crossword Puzzle have long been a cherished pastime, challenging our vocabulary and problem-solving skills. If you’re a puzzle enthusiast, you know the thrill of uncovering the perfect word that fits seamlessly into a grid. Today, we’re exploring a rather intriguing clue that’s recently been making rounds in various crossword puzzles.
The Intriguing Clue
The clue “Used as a campaign talking point” has piqued the curiosity of many. After scouring various sources and piecing together information, the answer that’s frequently coming up is “RANON.”
Understanding the Answer of Used as Campaign Talking Point Crossword Puzzle
But what does “RANON” mean, and why is it used as a campaign talking point? Let’s break it down:
- Campaign Talking Point:
- In political campaigns, talking points are key messages or positions that a candidate uses to communicate their platform and goals. These points are strategically crafted to resonate with voters and often become central themes in speeches and debates.
- RANON:
- While “RANON” may not be immediately recognizable, it likely stems from a specific context or thematic puzzle where the solver’s familiarity with the terminology or anagrams is tested.
Multiple Valid Answers
It’s crucial to note that crossword puzzles can sometimes have multiple valid answers, especially when clues are somewhat ambiguous or open to interpretation. This variability is part of what makes solving them so engaging and challenging.
for more information please visit “Muppet With Long Hooked Beak“
Tips for Solving Crossword Puzzles
If you find yourself stuck on a tricky clue like “Used as a campaign talking point,” here are a few tips to help you out:
- Context Matters:
- Consider the overall theme of the puzzle. Often, clues and answers are related to a central topic or pun.
- Think Synonymously:
- Think of synonyms or related phrases that might fit the clue. For example, other possible answers for campaign talking points could include “AGENDA” or “SLOGANS.”
- Check Crossings:
- Use the letters from the intersecting words to narrow down your options. Cross-referencing can often lead to that “aha” moment.
- Puzzle Specifics:
- Always keep the specific rules or guidelines of the puzzle creator in mind. Some puzzles may have unique twists or themes that influence the answers.
Also Check this: Unlocking the Secrets of Kanikama: A Guide for Beginners
Conclusion
While “RANON” seems to be the answer turning up for the clue “Used as Campaign Talking Point Crossword Puzzle” remember that crossword puzzles’ joy lies in their complexity and the multiple layers of meaning they can hold. Always double-check your answers and consider the puzzle’s specific context.
If you’re a puzzle enthusiast looking for more tips, tricks, and engaging content about your favorite pastime, stay tuned to our blog. We love sharing insights that make your problem-solving experience even more enjoyable.
Read More About Muppet With Long Hooked Beak
-
Travel2 years ago
NEW ZEALAND VISA FOR ISRAELI AND NORWEGIAN CITIZENS
-
Uncategorized2 years ago
AMERICAN VISA FOR NORWEGIAN AND JAPANESE CITIZENS
-
Health2 years ago
Health Benefits Of Watermelon
-
Lifestyle1 year ago
These Easy, Affordable Improvements Can Completely Transform Your Home
-
Technology1 month ago
Imagine a World Transformed by Technology and Innovation of 2023-1954
-
Lifestyle2 months ago
The Role of Door-to-Door Apps in Voter Engagement
-
Home Improvement9 months ago
11 Amazing Insights into Home Decor Gifts – Elevate Your Gifting Game
-
Business2 months ago
Metaphysical Stores Near Me